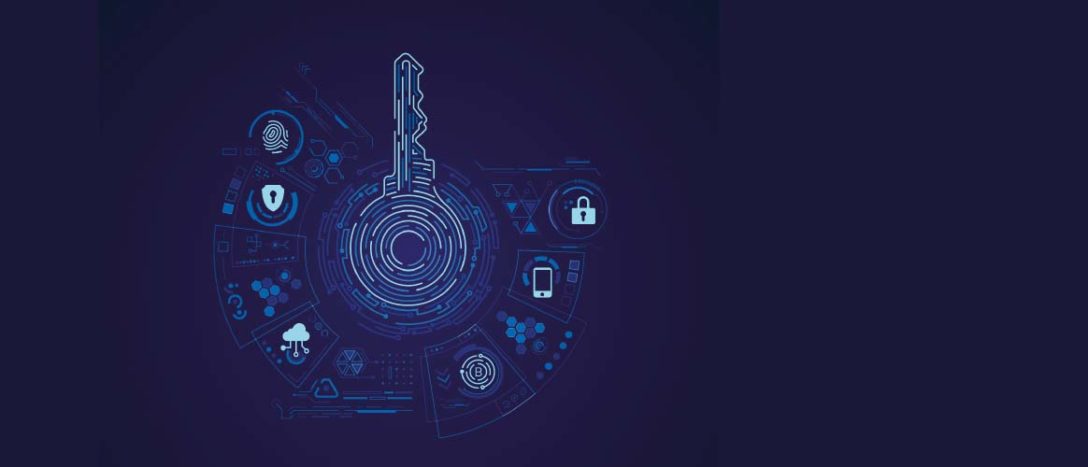
How AI could unlock opportunities in PE
Private equity has been slow to embrace the use of artificial intelligence, but data is dominant and those that fail to leverage its power may be left behind.
Private equity has been slow to embrace the use of artificial intelligence, but data is dominant and those that fail to leverage its power may be left behind.
Copyright PEI Media
Not for publication, email or dissemination